From lightbulb to launch:
How we built an evergreen AI foundation
All too often we get fixated on the rocket ship but forget about the launching pad. For AI to thrive within an enterprise healthcare environment, the focus needs to be on building the right foundation—one that can handle the whiplash of technological, regulatory, and organizational flux.
I think it’s safe to say that the healthcare industry is excited and hopeful about the promise of AI. Everyone—patients, clinicians, and health plans—are all on deck to reap the fruits of the next tech revolution.
Improved health outcomes, reduced costs, and better transparency. All of these possibilities are on the table, but how does a healthcare enterprise take the first step? Before we get tactical, here are a few hurdles you might run into throughout your AI journey.
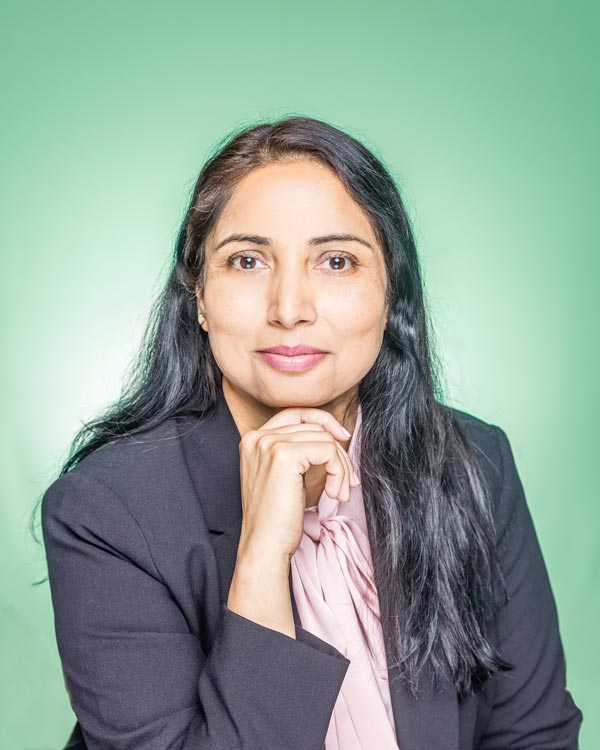
Tej Daruwalla
VP, Enterprise Data Platform
Stumbling blocks on the road to AI innovation in healthtech
Training an AI model is heavily reliant on trial and error. The model iteratively adjusts its parameters based on the feedback it receives during training. Ironically, launching a large-scale AI strategy across an enterprise is very similar. It’s one step forward, two steps back.
But if you can answer these challenges at the outset, it can be more like four steps forward.
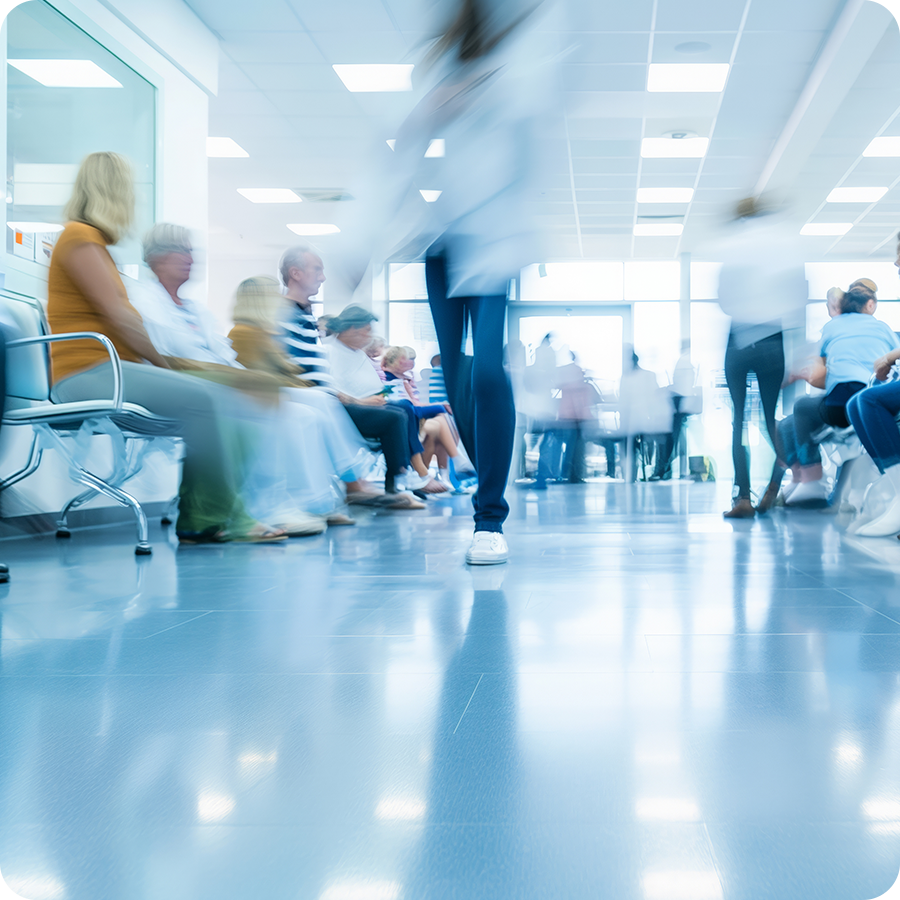
- Delays in care delivery: Authorizations that get caught in the system translate into poor patient experiences and unrealized care. It also leads to higher costs for payers and unpredictable revenue cycles for providers.
- Unnecessary administrative costs: Manual tasks, duplicated efforts, and communication breakdowns cause significant drops in efficiency. Providers are taken away from what matters and health plans get bogged down in a backlog of tasks.
- Inaccurate criteria: Without a consistent auth process, patient data can come from many sources. Outdated or misrepresented criteria can lead to faulty cost assessments, wrong diagnoses, and higher admin costs.
- Convoluted communications: Patients, providers, and administrators all need to be on the same page for effective care delivery. Without a reliable communication process, there can be too many channels to monitor. Faxes, mail, and phone calls to name a few.
- Ever-evolving regulations: An inconsistent, manual auth process poses many regulatory challenges when it comes to compliance. Without a streamlined digital footprint, keeping up with regulatory changes, compliance standards, and audit timelines is virtually impossible.
Misconceptions and expectations
When I talk to folks in healthcare about AI, many usually fall into two camps. Camp A believes that AI is a magic bullet. It can solve every organizational quandary; it knows no bounds; and all we have to do is build it.
Camp B thinks it’s all hype. The juice isn’t worth the squeeze; it’s far too risky, too expensive, and we should hold off until the odds are in our favor. Both perspectives are flawed. The challenge is to redirect expectations to the goldilocks zone in the middle.
Speed vs. responsibility
The balance between transformation and ethical responsibility weighs on healthcare innovators in a particular way. There’s a natural temptation to match the same speed as the AI news cycle. Adversely, there’s a growing mandate for health plans and providers to offer the best possible experiences technology affords.
The chosen few vs. everyone all at once
Organizations interested in forming a comprehensive AI strategy will face another pivotal choice. Who should steer the AI ship? What teams are responsible for development? Who decides which use-cases to pursue? Organizations without a proper operations structure will quickly devolve.
Solely relying on a small, specialized AI team can hinder widespread adoption and knowledge transfer across the organization. But total democratization—an all-at-once approach—can lead to indecision and chaos, especially amid high regulations and security mandates.
Data quality
Let’s say an organization has figured out all the above challenges. Without clean, high-quality data, AI systems cannot improve at scale. Due to healthcare’s legacy infrastructure, this can be a costly step backward for some. The “garbage in, garbage out” reality of AI development needs to be considered (and reconciled) as early as possible.
Finding the friction: How we uncovered challenges in the auth process
After analyzing data, reviewing provider feedback, and sifting through patient surveys, we discovered a decent amount of friction holding back the authorization process. For patients, providers, and health plans, an inefficient auth process means:
• Delays in care delivery: Authorizations that get caught in the system translate into poor patient experiences and unrealized care. It also leads to higher costs for payers and unpredictable revenue cycles for providers.
• Unnecessary administrative costs: Manual tasks, duplicated efforts, and communication breakdowns cause significant drops in efficiency. Providers are taken away from what matters and health plans get bogged down in a backlog of tasks.
• Inaccurate criteria: Without a consistent auth process, patient data can come from many sources. Outdated or misrepresented criteria can lead to faulty cost assessments, wrong diagnoses, and higher admin costs.
•Convoluted communications: Patients, providers, and administrators all need to be on the same page for effective care delivery. Without a reliable communication process, there can be too many channels to monitor. Faxes, mail, and phone calls to name a few.
•Ever-evolving regulations: An inconsistent, manual auth process poses many regulatory challenges when it comes to compliance. Without a streamlined digital footprint, keeping up with regulatory changes, compliance standards, and audit timelines is virtually impossible.
For many in the industry, these challenges aren’t exactly surprising. According to McKinsey, “...clinical staff must devote considerable time to reviewing [authorization] requests. Meanwhile, doctors and staff report spending 13 hours per week on [authorizations]; many clinicians believe it undermines their clinical judgment and can inhibit timely care.”
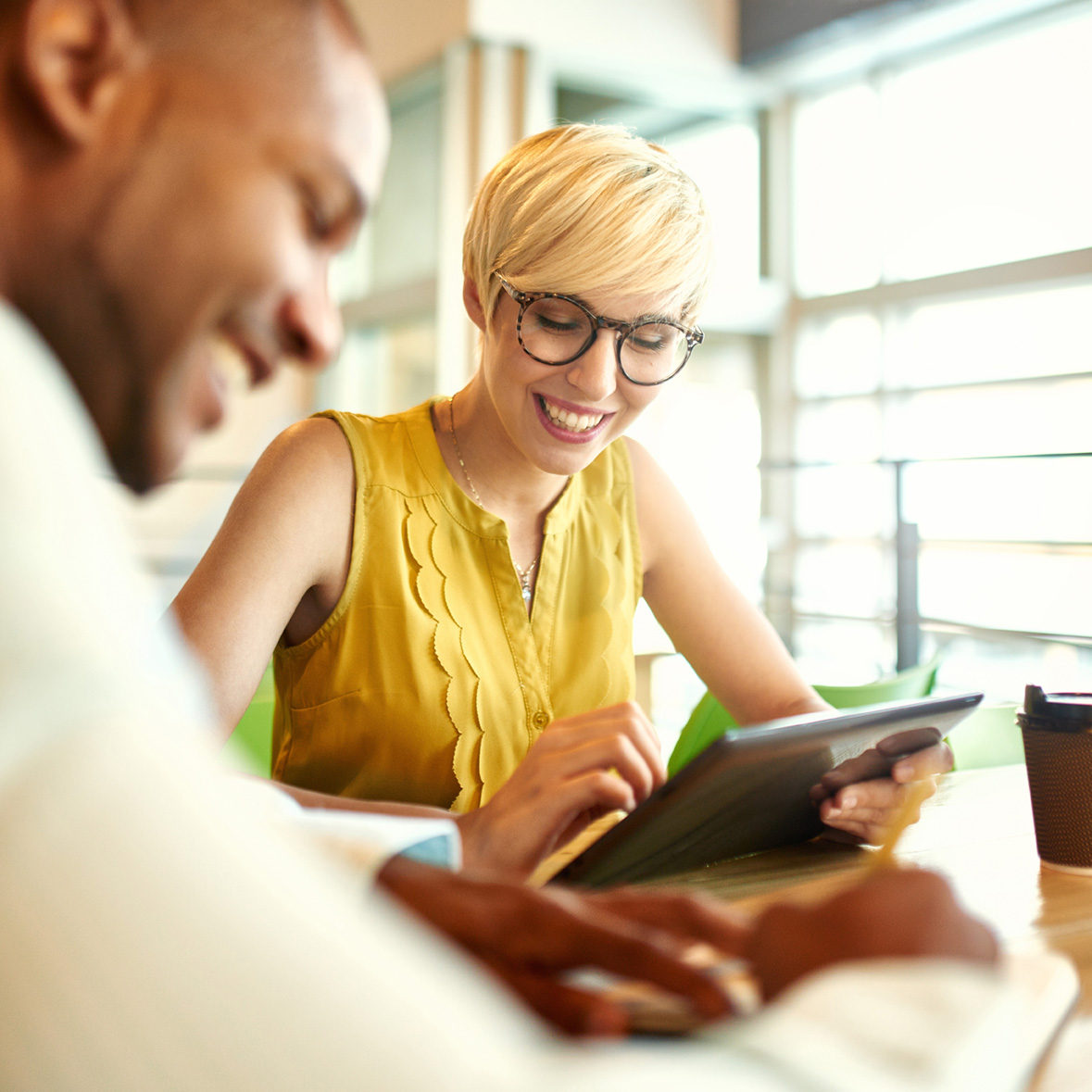
How to eat an AI elephant:
Three tactical bites for the healthcare enterprise
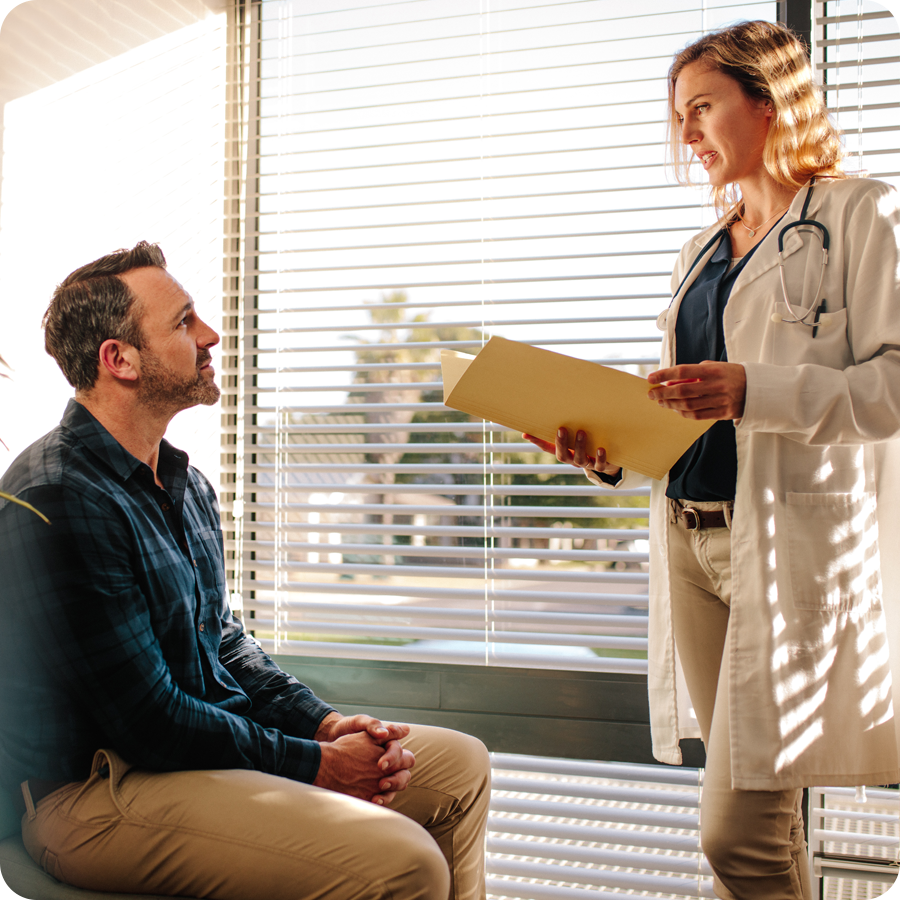
1. Align, educate, and engage
At the very beginning of our AI journey, there were many misconceptions and expectations from stakeholders. Folks were worried about job displacement, reliability, and ROI. These concerns are all valid. But without the proper knowledge, reaching a level of company-wide alignment can put a damper on progress.
The first and foremost step to rolling out a successful AI motion is to gain buy-in from executive leadership. This requires a deliberate, phased approach involving education, proof of concepts, and pilots. In short, you need to demonstrate the value of AI through tangible results. Additionally, there needs to be a concerted effort around general knowledge and education. Deep dives about automation vs. AI; traditional AI vs. Generative AI; and the business potential were pivotal in garnering trust. And this isn’t just for executive leaders.
At some point your entire organization will need to be familiar with the foundational principles of AI. There needs to be a radical culture shift in the mindset of your people. Without this component, adoption, change management, and user efficiencies will depreciate.
Six months. That’s about how long it can take for an organization’s newly minted AI strategy to become outdated. For those of us in healthcare, the challenge is even greater. A highly regulated environment, legacy infrastructures, and misaligned priorities necessitate the need for a launch pad—not a rocket ship.
These three tactics are best viewed as the cornerstones of your AI foundation. Yes, they’re actionable, but view them as long-term checkpoints.
1. Align, educate, and engage
At the very beginning of our AI journey, there were many misconceptions and expectations from stakeholders. Folks were worried about job displacement, reliability, and ROI. These concerns are all valid. But without the proper knowledge, reaching a level of company-wide alignment can put a damper on progress.
The first and foremost step to rolling out a successful AI motion is to gain buy-in from executive leadership. This requires a deliberate, phased approach involving education, proof of concepts, and pilots. In short, you need to demonstrate the value of AI through tangible results. Additionally, there needs to be a concerted effort around general knowledge and education. Deep dives about automation vs. AI; traditional AI vs. Generative AI; and the business potential were pivotal in garnering trust. And this isn’t just for executive leaders.
At some point your entire organization will need to be familiar with the foundational principles of AI. There needs to be a radical culture shift in the mindset of your people. Without this component, adoption, change management, and user efficiencies will depreciate.
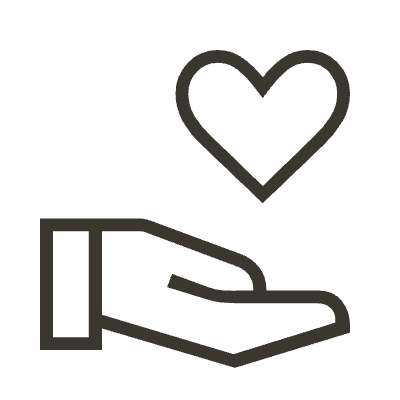
2. Build a responsible AI framework
During the early days of planning and development, we took a very thoughtful approach to AI. We intentionally prioritized security, compliance, and ethical responsibility over speed-to-market. Our governing principle—our AI North Star—is to never put members, patients, or providers in any kind of jeopardy. We do this in three ways:
3. Identify, prioritize, and pilot the right use cases
The beauty of building a launching pad instead of a rocket ship is the ability to build multiple rocket ships with efficiency and predictability. This is the core principle behind enGen’s AI Foundry—our homegrown framework for an intentional, long-term AI strategy. The goal is to curate a backlog of high-value use cases. The Foundry follows a four-phase process:
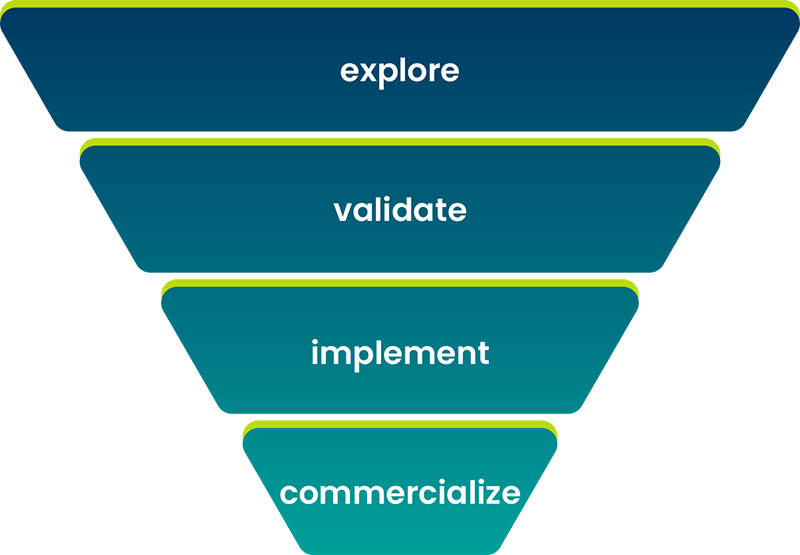
1. Explore:
We begin with a company-wide, unfiltered brainstorming session to gather potential AI applications. Leaders across departments are looped in. This broad participation sparks wider adoption, builds internal enthusiasm, and generates greater impact overall. Every suggestion, big or small, is captured in this vital step.
2. Validate:
For promising ideas, we carefully develop robust business cases, evaluating ROI, potential revenue growth, and cost reduction opportunities. Financial viability is paramount—we emphasize value demonstration to stakeholders and leadership to gain buy-in. Everything is mapped to growth goals—whether it’s an internal or commercialized use case.
3. Implement:
Successful AI projects require lots of pilot testing and iteration. The goal is to measure efficacy while gathering crucial data that serves as a living proof-of-concept. Our first pilot focused on internal product engineering (our bread and butter) and lasted about 10 weeks. We leaned on a small team of skilled, curious product experts to create a walled garden and get creative.
4. Commercialize:
Based on successful piloting, proven projects are brought to the Executive Steering Committee for review. We then move to company-wide implementation with the intention of achieving the projected ROI. Performance measurement is continuous.
Six months. That’s about how long it can take for an organization’s newly minted AI strategy to become outdated.
Closing Thoughts: The TL;DR version
The human mind tends to overcome limited paths with ease. Shortcuts, hacks, and innovation always cultivate a new way forward. But in my opinion the challenge is greater when there are limitless possibilities. And that’s what AI brings to the table. Whichever path you choose, try to find the constant, evergreen principals:
Focus on business value:
AI adoption should not be driven by tech glitz and glamor but by quantifiable business results. Try to understand the greatest business need or direction and strap AI to the back of these pursuits. Use data-driven outcomes as proof of concepts.
Champion internal buy-in:
At some level, everyone has to agree on the direction of AI. The long-term goal needs to serve as the anchor to provide stability for the short-term turbulence. In other words, leadership needs to agree on the “why” before moving to the “how.” The effect is a streamlined path that reduces speculation and hesitancy.
Embrace gradual implementation:
Slow is smooth and smooth is fast. A structured phased approach minimizes risks and promotes scalable AI growth. Gradually widening an organization's experience reduces the risk of both technical and organizational setbacks.
In hindsight, the closing thoughts above seem so simple and obvious, but in reality, they’re hard-won lessons learned through experience and experimentation. So, if you need to choose a path—any path—consider this one.
Remember: stay the course and don’t try to match pace with the headlines.